联邦分析赋能的频繁模式挖掘用于去中心化的Web 3.0应用
Authors:
Zibo Wang, Yifei Zhu, Dan Wang, Zhu Han
Abstract:
The emerging Web 3.0 paradigm aims to decentralize existing web services, enabling desirable properties such as transparency, incentives, and privacy preservation. However, current Web 3.0 applications supported by blockchain infrastructure still cannot support complex data analytics tasks in a scalable and privacy-preserving way. This paper introduces the emerging federated analytics (FA) paradigm into the realm of Web 3.0 services, enabling data to stay local while still contributing to complex web analytics tasks in a privacy-preserving way. We propose FedWeb, a tailored FA design for important frequent pattern mining tasks in Web 3.0. FedWeb remarkably reduces the number of required participating data owners to support privacy-preserving Web 3.0 data analytics based on a novel distributed differential privacy technique. The correctness of mining results is guaranteed by a theoretically rigid candidate filtering scheme based on Hoeffding’s inequality and Chebychev’s inequality. Two response budget saving solutions are proposed to further reduce participating data owners. Experiments on three representative Web 3.0 scenarios show that FedWeb can improve data utility by ~25.3% and reduce the participating data owners by ~98.4%.
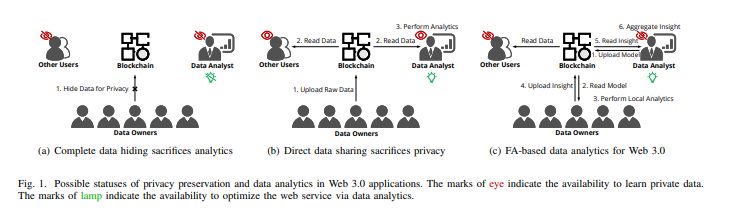
摘要中文:
新兴的Web 3.0范式旨在分散现有的Web服务,实现透明性、激励和隐私保护等理想属性。然而,由区块链基础设施支持的当前Web 3.0应用仍然不能以可扩展和隐私保护的方式支持复杂的数据分析任务。本文将新兴的联合分析(FA)范式引入Web 3.0服务领域,使数据能够保持在本地,同时仍然以保护隐私的方式为复杂的Web分析任务做出贡献。我们提出了FedWeb,一个为Web 3.0中重要的频繁模式挖掘任务量身定制的FA设计。FedWeb显著减少了支持基于新型分布式差分隐私技术的隐私保护Web 3.0数据分析所需的参与数据所有者的数量。基于Hoeffding不等式和Chebychev不等式的理论上严格的候选过滤方案保证了挖掘结果的正确性。提出了两种节省响应预算的解决方案,以进一步减少参与的数据所有者。在三个有代表性的Web 3.0场景上的实验表明,FedWeb可以提高数据效用约25.3%,减少参与数据所有者约98.4%。
研究内容:
- 引言:介绍了Web 3.0与联合分析的结合,以及当前Web 3.0在隐私保护数据分析方面的不足。
- 预备知识:讨论了Web 3.0相关的隐私标准,包括差分隐私(Differential Privacy, DP)。
- 威胁模型和问题表述:定义了数据分析师和数据所有者之间的半诚实模型,并提出了隐私保护频繁模式挖掘的问题。
- 隐私保护FPM设计:详细描述了面向Web 3.0的FA基础隐私保护FPM方案FedWeb。
- 评估:在不同的Web服务场景中评估FedWeb,并与现有本地隐私FPM解决方案进行比较。
- 相关工作:讨论了Web 3.0数据分析和FA的相关研究。
- 结论:总结了本文的主要贡献和FedWeb方案的优势。
论文pdf 链接:https://arxiv.org/pdf/2402.09736